
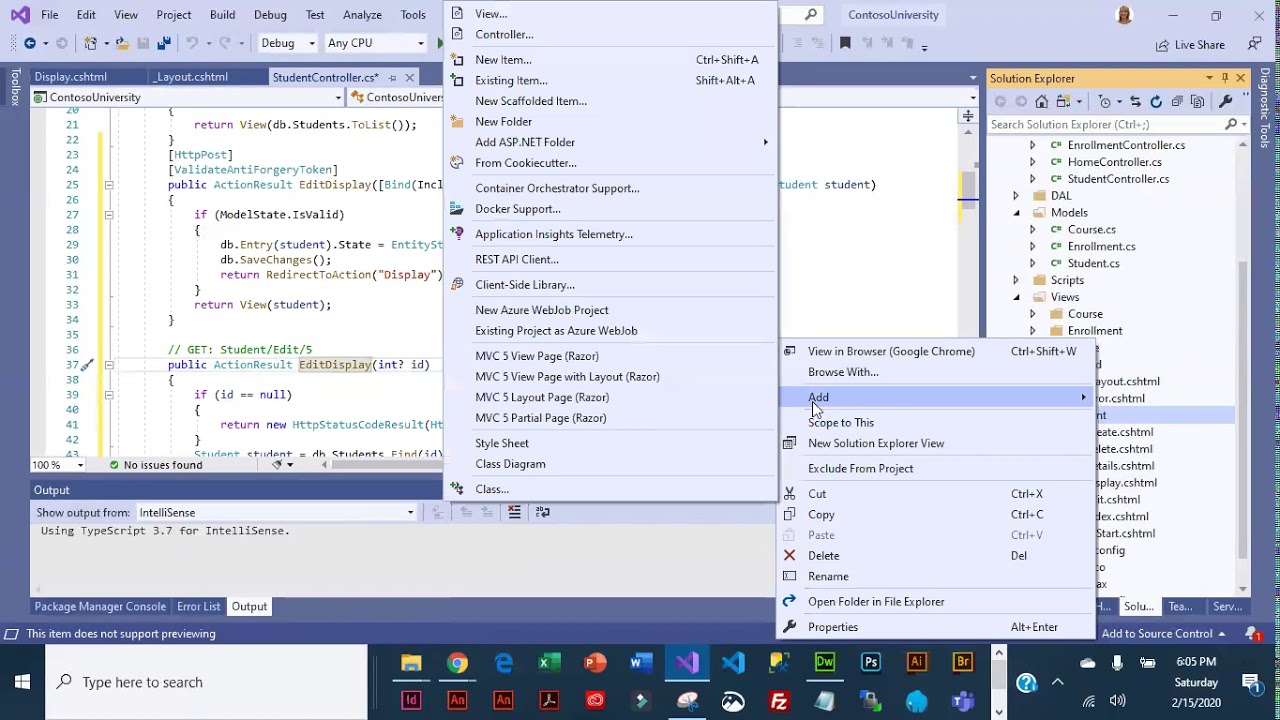
To ensure the accuracy and quality of the annotations, the Innodata team utilized a double-blind pass process, in which two different annotators provide annotations and an adjudicator provides a judgement on any discrepancies between the annotations. This process of annotating utterances and assigning labels from a taxonomy allowed the chatbot to understand customer intent from incoming messaging and provide relevant and accurate responses. To achieve this, the Innodata team annotated incoming chatbot messages for any mention of specific hotels, occurrences of locations (including cities, regions, districts, and addresses), and categorized the intent of the utterances based on their subjective interpretation of the message. To reach the seamless performance expected by the travel aggregator and its customers, the chatbot needed to be trained for many utterances per intent in English, Chinese, and French.
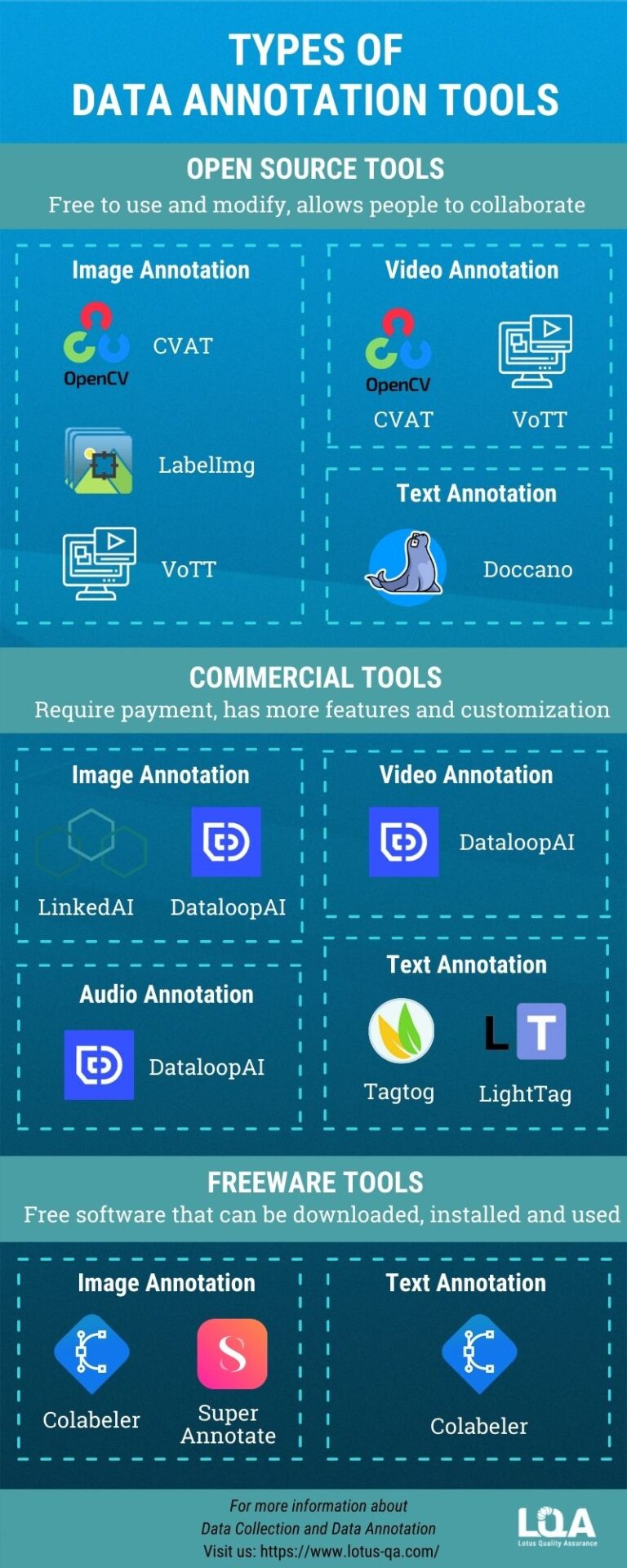
Leading travel aggregator and booking engine required highly accurate datasets for a booking assistant bot that operates in multiple languages. This pristine data, along with the risk taxonomy provided, helped train and improve the model performance.

The leading global financial services company's risk assessment platform received a large annotated dataset of the highest quality based on thousands of relevant articles. They then identified risks within each article and assigned a risk category and level based on the agreed-upon taxonomy. The platform and annotators labeled the risks associated with events, named individuals, and named companies within each article. Finally, our quality assurance specialist reviewed and resolved any discrepancies. With high-quality, human-powered data annotation, companies can build and improve AI implementations. Training data must be properly categorized and annotated for a specific use case. Then experts did a round of annotations to ensure accuracy and reviewed any low confidence annotations. Data annotation is the categorization and labeling of data for AI applications. The articles were first run through Innodata's proprietary text annotation platform, which completed an auto annotation. To bolster speed and ensure high-quality annotations throughout the articles, Innodata employed a combination of humans-in-the-loop and ML-enhanced technology. Innodata's subject matter experts created a taxonomy focused on model-relevant risk categories and risk stages. Global financial services firm required the annotation of technical financial documents to train its AI platform to conduct risk assessments for investment portfolios.
